Liquid AI, a new MIT spinoff, to Build a New Type of AI
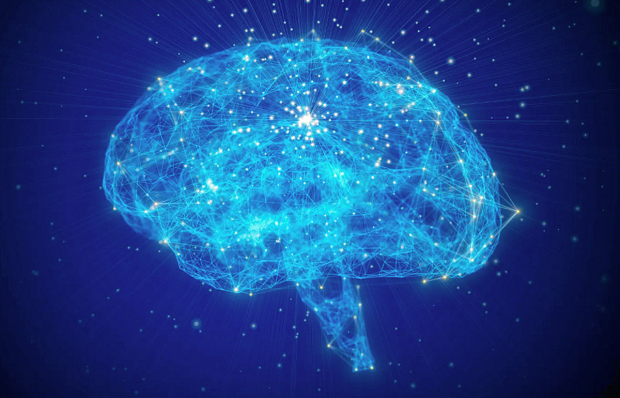
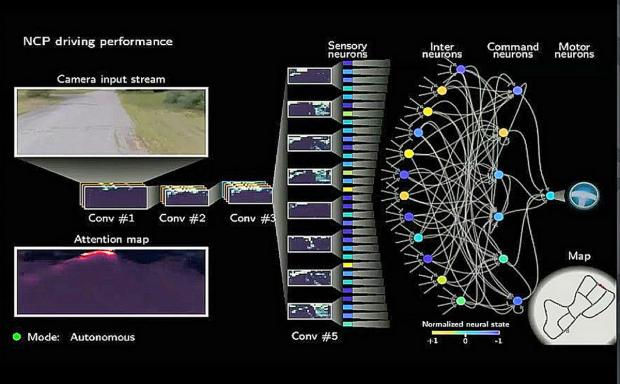
An MIT spinoff co-founded by robotics luminary Daniela Rus aims to build general-purpose AI systems powered by a relatively new type of AI model called a liquid neural network. Liquid AI announced that it has raised $37.5 million from VCs and organizations including OSS Capital, PagsGroup, WordPress parent company Automattic, Samsung Next, Bold Capital Partners and ISAI Cap Venture, as well as angel investors like GitHub co-founder Tom Preston Werner, Shopify co-founder Tobias Lütke and Red Hat co-founder Bob Young. The tranche values Liquid AI at $303 million post-money. Joining Rus on the founding Liquid AI team are Ramin Hasani (CEO), Mathias Lechner (CTO) and Alexander Amini (chief scientific officer). Hasani was previously the principal AI scientist at Vanguard before joining MIT as a postdoctoral associate and research associate, while Lechner and Amini are longtime MIT researchers, having contributed — along with Hasani and Rus — to the invention of liquid neural networks. “The idea was invented originally at the Vienna University of Technology, Austria at Professor Radu Grosu’s lab, where I completed my Ph.D. and Mathias Lechner his master’s degree,” Hasani told TechCrunch in an email interview. “The work then got refined and scaled at Rus’ lab at MIT CSAIL, where Amini and Rus joined Mathias and I.”
Liquid neural networks consist of “neurons” governed by equations that predict each individual neuron’s behavior over time, like most other modern model architectures. The “liquid” bit in the term “liquid neural networks” refers to the architecture’s flexibility; inspired by the “brains” of roundworms, not only are liquid neural networks much smaller than traditional AI models, but they require far less compute power to run. Liquid neural networks adapt their parameters for “success” over time. The networks consider sequences of data as opposed to the isolated slices or snapshots most models process and adjust the exchange of signals between their neurons dynamically. These qualities let liquid neural networks deal with shifts in their surroundings and circumstances even if they weren’t trained to anticipate these shifts, such as changing weather conditions in the context of self-driving. Watch a video, here. For more details, visit Techcrunch.com. Image Credits: MIT CSAIL, dem10 / Getty Images.